Ever since the launch of DiVoMiner® platform, it has empowered many researchers in conducting their content analysis in social sciences. One key aspect of content analysis is the coding of data, which refers to the analytical process of transforming collected data to a set of meaningful and cohesive categories, in order to bring out the value and meaning of the data (Allen, 2017).
In the “big data” context, the information obtained from Internet-based sources is much more voluminous, dynamic and unstructured, such as social media post and comments on the digital platforms (Lazer et al., 2009; Shah, Cappella, & Neuman, 2015). Therefore, a data-driven “AI-assisted content analysis” approach is proposed by our team to support researchers on their content analysis in an efficient and effective way, aiming to save 80% of their time on repetitive work like coding. So far, multiple researchers have used the machine/manual coding module on DiVoMiner®, and published their paper on SCI-E (Patient Education and Counselling, Journal of Medical Internet research, International Journal of Environmental research and Public Health) and SSCI journals (Public Relations Review, Chinese Journal of Communication). Here are some examples:
- “The embedded software in DiVoMiner provides automated content analysis while validation and reliability check are also functional. Thus, machine computational method contributes valuable clues for examining news selection in a more efficient, effective, and transparent process.”
“DiVoMiner assisted in the implementation of the coding taxonomy to measure the concept of misinformation. The process involves pilot coding, subsequent modification of the coding scheme, and double coding adapted from earlier studies.”
Chang, A. (2020). Misinformation from Web-based News Media? Computational Analysis of Metabolic Disease Burden for Chinese. In: van Duijn, M., Preuss, M., Spaiser, V., Takes, F., Verberne, S. (eds) Disinformation in Open Online Media. MISDOOM 2020. Lecture Notes in Computer Science (12259). Springer, Cham. https://doi.org/10.1007/978-3-030-61841-4_4
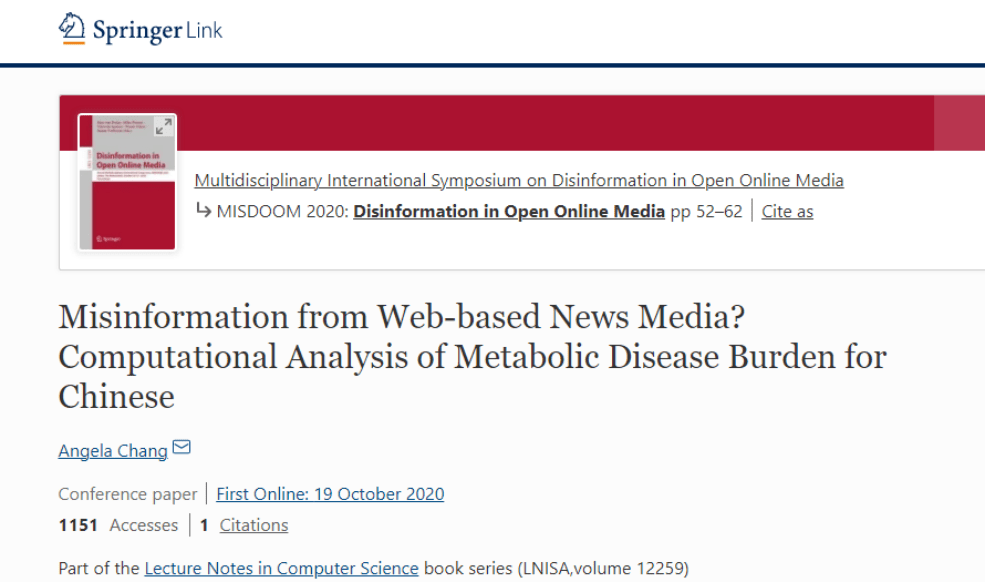
- The semantic machine learning model having been introduced into DiVoMiner, the textual mining and analysis platform can not only be used for manual coding, but also for machine learning coding, multidimensional analysis, analysis of the correlation between multiple variables, cross analysis, regression analysis, statistical verification, and the creation of word clouds.
Tu, S., Lu, L. Y., Hsieh, C., & Wu, C. (2021). A New Internet Public Opinion Evaluation Model: A Case Study of Public Opinions on COVID-19 in Taiwan. International Journal of Big Data and Analytics in Healthcare (IJBDAH), 6(2), 1-17. http://doi.org/10.4018/IJBDAH.287603
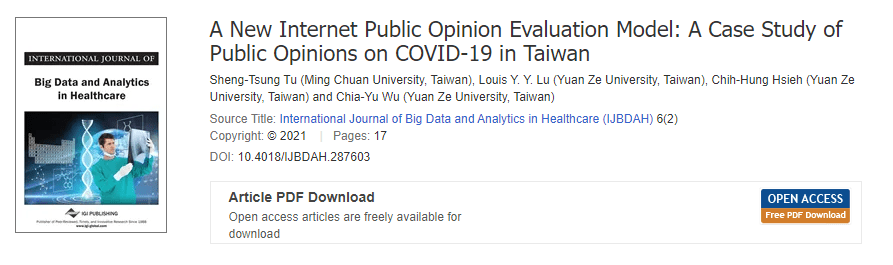
- “Specifically, based on the internal rules of mining text, DiVoMiner guided the machine to automatically label obesity and its related keywords. The classification results of algorithm coding generated by DiVoMiner were obtained simultaneously.”
Chang, A., Schulz, P. J., Jiao, W., & Liu, M. T. (2021). Obesity-Related Communication in Digital Chinese News From Mainland China, Hong Kong, and Taiwan: Automated Content Analysis. JMIR public health and surveillance, 7(11), e26660. https://doi.org/10.2196/26660
- “We combined machine coding with manual coding in the encoding process by using DiVoMiner.”
“DiVoMiner uses machine-learning and manual-correction coding methods, enabling the entire process of content analysis to be performed online. First, two coders independently coded 150 posts. Upon completion of manual coding, DiVoMiner was used to code the remaining posts.”
Zhou, F., Zhang, W., Cai, H., & Cao, Y. (2021). Portrayals of 2v, 4v and 9vHPV vaccines on Chinese social media: a content analysis of hot posts on Sina Weibo. Human vaccines & immunotherapeutics, 17(11), 4433–4441. https://doi.org/10.1080/21645515.2021.1971016
- “The platform of DivoMiner integrating artificial intelligence and automatic coding provides reliable and powerful research execution and management.”
“The computational platform combined automatic content analysis while considering traditional content analysis procedures. To investigate how the COVID-19 vaccine controversy spread and influenced judgments of vaccine risk, a codebook was developed and tested in DivoMiner.”
Chang, A.,&Ho, M..(2022).Heightening fake information and misinformation around COVID-19 vaccine controversy by examining super-spreaders’ lies. Fake Information and Global Communication. https://repository.um.edu.mo/handle/10692/111329
References:
Allen, M. (Ed.) (2017). The sage encyclopedia of communication research methods. (Vols. 1-4). SAGE Publications, Inc, https://dx.doi.org/10.4135/9781483381411
Krippendorff, K. (1980). Content analysis: An introduction to its methodology. Beverly Hills, CA: Sage.
Lazer, D., Pentland, A., Adamic, L., Aral, S., Barabasi, A. L., Brewer, D., Christakis, N., Contractor, N., Fowler, J., Gutmann, M., Jebara, T., King, G., Macy, M., Roy, D., & Van Alstyne, M. (2009). Social science. Computational social science. Science (New York, N.Y.), 323(5915), 721–723. https://doi.org/10.1126/science.1167742
Neuendorf, K. A. (2002). The content analysis guidebook. Thousand Oaks, CA: Sage.
Shah, D. V., Cappella, J. N., & Neuman, W. R. (2015). Big data, digital media, and computational social science: Possibilities and perils. Annals of the American Academy of Political and Social Science, 659(1), 6–13. https://doi.org/10.1177/0002716215572084