DiVoMiner® is an AI-aided content analysis platform (ACA) for processing textual data with artificial intelligence and content analysis approach. Our platform features machine learning and manual refinement mechanism, to effectively process data in a one-stop fashion. Researchers can effectively complete the process of database building, sampling, inter-coder reliability test, coding, quality control, statistical analysis, and data visualization. The platform provides powerful and flexible team collaboration and management functions. It is a web mining and text analysis platform that fulfills both practical and academic requirements.
The design concept of DiVoMiner® platform is online content analysis assisted by Artificial Intelligence (AI). In the context of massive and complex data, making analysis can be difficult from the scratch. Therefore, at the beginning of the research, it is necessary to structure the online and offline data during collection, to form the database. Secondly, utilizing the computer algorithm with methods like web mining, machine learning, and semantic network analysis to quickly obtain preliminary data results. Then you can have an overview of the data and discover unknown patterns in details. However, in most research scenarios, only using machine analysis may not be able to achieve sufficient research depth. Therefore, it is necessary to further explore the meaning and insights of the patterns. In this case, online content analysis can be used, through machine coding and manual coding. An integration of machine learning coding with human intelligence can ultimately perform deep mining. In doing so, we can achieve “Data In, Value Out”.
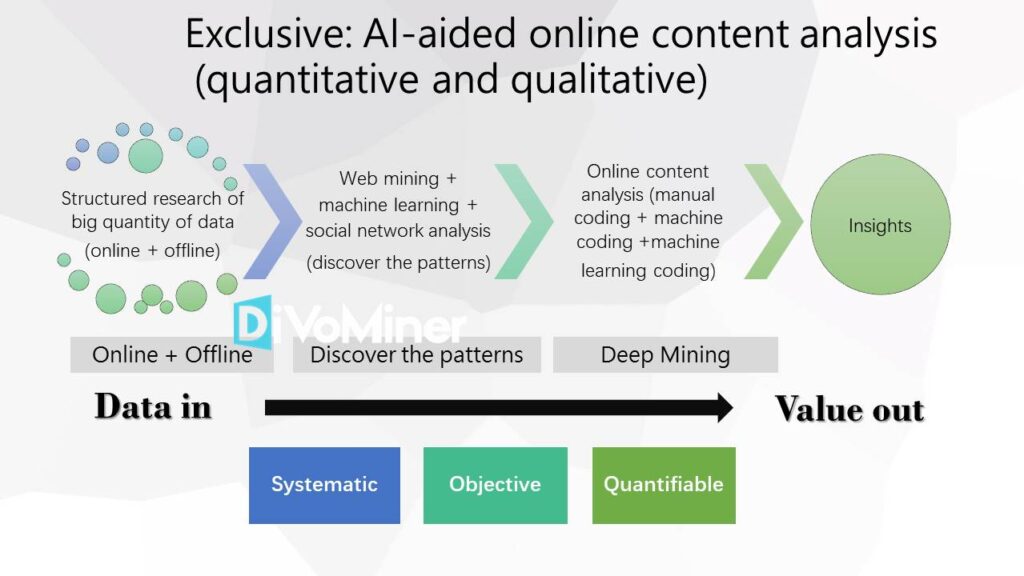
DiVoMiner® integrates the systematic, objective and quantifiable research methods throughout the whole research process. The operation procedures are divided into four phases: preparation, exploration, online content analysis (coding and quality monitoring), and result presentation.
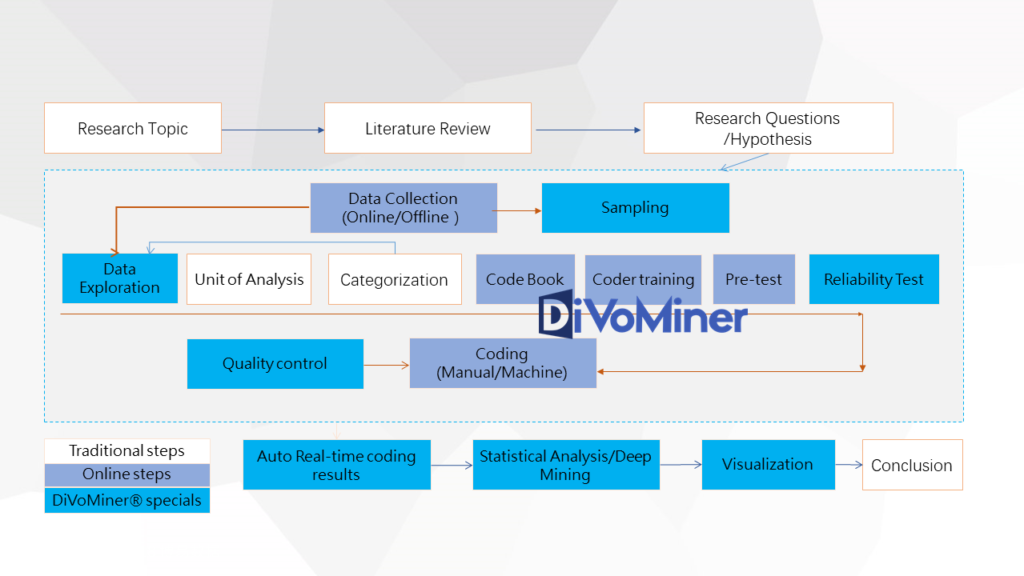
- 1. Preparation:
To prepare a database, identify different types of data sources and establish sub-databases accordingly for different sources. For example, the data formats of historical documents and social media are different, so users can upload different types of data with different formats to the corresponding sub-database for the database building.
For database management, DiVoMiner® has four types of data pools for different functions:
Coding Pool: User can manage all the uploaded data in the coding pool, and data in different formats are placed in different sub-databases. The data fields (variables) can be shared between different sub-database. Subsequent data analysis such as data exploration, online content analysis, statistical analysis, and further in-depth data visualization are all based on the data in the coding pool.
Recycle Bin: After data cleaning, the deleted data is put in the pool of Recycle Bin.
Pretest Pool: Designed for coding pretest, it is required that coders code the same data in the pretest pool, in order to calculate the inter-coder reliability for content analysis. When the inter-coder reliability reaches an acceptable level, formal coding can start.
Sampling Pool: If data sampling is needed, a sampling pool can be established with a portion of the data randomly or through other sampling methods, to facilitate the research project.
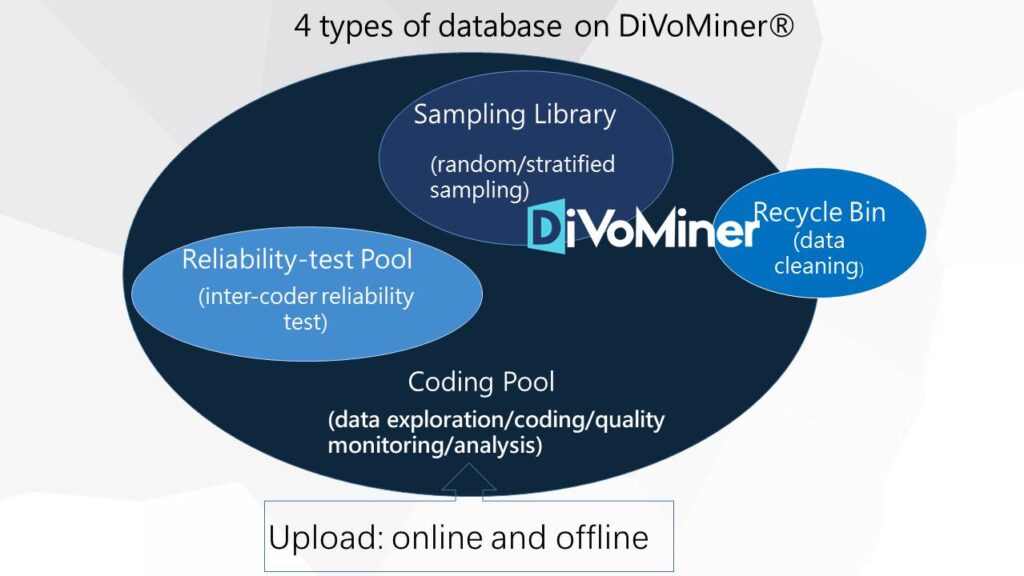
- 2. Data exploration:
Data exploration is the first step of data analysis used to explore and visualize data with machine analysis methods such as network mining or algorithm models. It is an initial step to quickly analyze and overview the data. The platform can present the overall trend of the data in the form of a time series chart, as well as the major themes of the content in the form of a word cloud diagram.
- 3. Online content analysis:
Coding categories are the basis of content analysis. To a certain extent, the quality of category construction determines the quality of content analysis. Researchers design coding categories, and coders read text and code/classify them. The coding category list is also called the codebook, similar to the questionnaire in a survey.
For text coding, both machine coding and manual coding can be used. For categories with strong objectivity, such as the name of a person and organization, machine coding can be applied. At this level, machine coding is fast, highly accurate and efficient. For categories with strong subjectivity, such as intention and attitude, the accuracy of machine coding may be compromised, and manual coding is recommended.
For quality control, DiVoMiner® provides real-time data monitoring, which can track the performance of each coder and the coding results at any time, and offer a convenient way to correct coding results.
- 4. Data visualization:
After completing the content analysis, it is the data analysis phase. On the platform, you can have an overview of the frequency of a single variable (“Coding Results” section). The platform also support customized data analysis charts (“Statistical Analysis” section) for users. Through simple drag-and-drop operations, users can generate statistical analysis charts and adjust the visualization effects, according to their research needs.